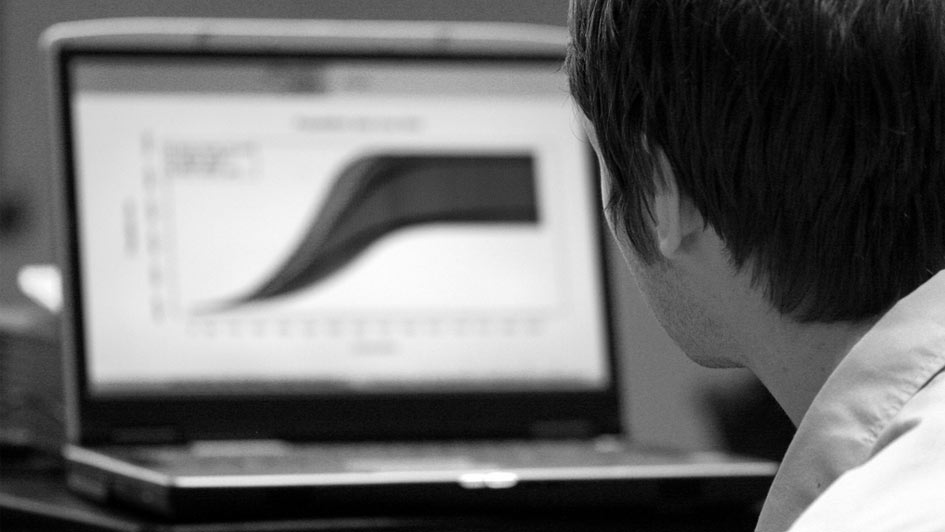
“Big Data” sounds like what it purports to be: “Big.” Advocates claim that it gives market researchers capabilities we never dreamed of. It is not simply part of the future; it owns the future and will supplant other forms of data collection. “Big” companies are making use of these kinds of data. You better too or you’ll soon become an anachronism. As with anything new and revolutionary, some of the claims are overblown. There are also criticisms that are warranted and criticisms that are misplaced. In other words, there’s a lot of confusion in marektingland about “Big Data.” Big data leverages the digital storage of information. We have at our disposal an enormous amount of data about an enormous number of people. We can know what they search for, what they purchase, what they read about. We can, in many ways, know how they spend their time, sometimes in real time. And our high-speed computers can deal with all of these data. There is virtually no limit to the amount of information we can analyze. Further, because we have so much information on so many people, we can segment populations in an almost infinite number of ways and look at relationships we never could have studied before. For example, we can, in principle, study divorced soccer moms with two kids who shop at Walmart and drive all-wheel drive SUVs. Or we can relate the shoe-buying behavior of this population to a host of variables. We can examine myriad variables to predict specific behaviors in very specific populations. We now have the numbers and the number-crunching technology.
Strengths of Big Data
Big data is about data and there are general rules that cover working with data. All measurements and, therefore, all data are flawed. Each piece of data (datum) contains error. The more data you collect, the closer you get to the actual value of the variable being studied because error tends to be random so that the value of the data tends to become closer to the true value the more data you collect. Think of flipping a coin. Let’s say you do it ten times and get six heads. Can you conclude that the coin is biased to heads? Of course not. But let’s say you flipped it 1,000,000 times and got 600,000 heads. Now can you conclude that it is biased? Yes. This is one major principle of data collection favoring big data.
READ MORE: On the Venn Diagram of Brand Value, Meaning and Design Must Merge
Here is another thing big data offers. When we predict an outcome using a lot of possible predictors, the statistical formula weights each predictor to maximize the prediction. When there are not a lot of data, this tends to be arbitrary and unreliable; it’s not to be trusted. Because of the numbers in big data, the weights tend to be reliable and we can test if the formula works by trying again with another huge data set. We can gain insights that we never could before. One final characteristic of big data is what I would call blind empiricism. We need not have a hypothesis. We can just throw every variable into the statistical mix and see how they predict the outcome. What we have is what advocates of big data call the What, instead of the Why. We don’t know why these variables, weighted in this way, predict what we are interested in, but we do know that they do. If we are marketers, we may not care. If I can predict the purchase of shoes with a host of variables, I may not care why they predict it.
Issues with Big Data
But all is not sweetness and light. There are issues around big data, as well. A major one revolves around the lack of theory. Some tout it as a strength of big data that we need not have a hypothesis. Let the data fall where they may and predict stuff. There is some truth to this, but here is the flip side. We can only analyze information we have. There could be critical variables that strongly predict our outcome. If we don’t collect them, we’ll never know. Only theories and hypotheses can tell us where to look for such things. Big data cannot. When we create formulas with big data, they tend to be heavy with demographics and search behaviors. So it may seem that they predict all. They don’t. For example, we’ll miss personality variables. We’ll miss unconscious variables. We don’t routinely collect those so we can’t throw them into our statistical formulas. We end up with a skewed view of purchase (or any other kind of) behavior. Oh, we’ll have a correlation – and it’ll be predictive. But it could miss what is critical.
READ MORE: Why Did You Say You Bought This?
And, then there is something that should be obvious, but is not. No matter how many variables we have, we have to predict something. We need an outcome. Do we want to predict shoe buying? What kinds of shoes? Men’s? Women’s? Children’s? Specialty footwear? Seasonal? No matter what, we have to pick an outcome. This is not always as easy as it seems. I mentioned divorced soccer moms with two kids who shop at Walmart and drive four-wheel drive vehicles. We can certainly look at them. But why? Outcome is a problem many companies are running into as they try to leverage big data. What do they want to predict? Without a clear outcome variable, it’s all nonsense. The human element is essential. Finally, as in any research enterprise, the data do not interpret themselves. Data are interpreted by scientists, marketers or other humans. Scientists do not usually argue over the data. They argue over interpreting data, which only humans can. To some degree this sets apart the hack from the creative genius. All physicists had access to the data Einstein had. More, in fact, as he was working in a patent office. But Einstein was able to integrate and interpret the data in radically novel ways that led to theories and hypotheses and, therefore, to the collection of very specific data that proved him right. Big data cannot do this.
Whither Big Data?
If marketers expect big data to solve their problems, they will be disappointed. Someone must decide what to predict and what kinds of data to collect to make the best predictions. Then, someone must interpret the data. Once these problems are addressed, big data can be a revolutionary way to help, but it will never answer these questions on its own. That is why it is touted and criticized, and is creating such confusion among researchers today. In sum, big data is a wonderful new tool. It enables complex data analyses never before possible. It can lead to all sorts of unexpected findings. But it cannot analyze data that were not collected, it cannot generate hypotheses to decide what kinds of data to collect and it cannot interpret data. It is but a tool, not a panacea.
Photo credit: Nic McPhee / Foter